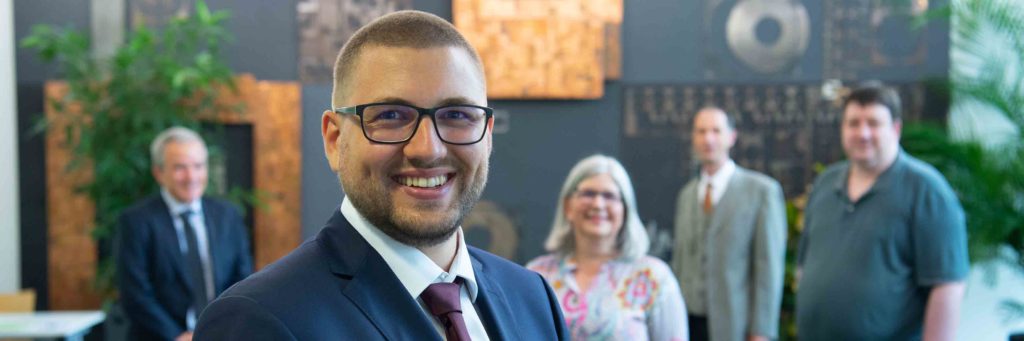
Jascha Kolberg defended his Ph.D. Thesis on Security Enhancement and Privacy Protection for Biometric Systems
Jascha Kolberg successfully defended his Ph.D. thesis entitled „Security Enhancement and Privacy Protection for Biometric Systems“ on July 8th 2021. The event itself was done in a hybrid format with a few people being allowed to attend in person and all others had the opportunity to follow the live stream remotely. The examining committee members were Prof. Dr. Ralf Dörner, Prof. Dr. Raymond Veldhuis, and Prof. Dr. Andreas Uhl. The thesis was carried out at the PZAI (Promotionszentrum Angewandte Informatik) at the University of Applied Sciences Darmstadt and was supervised by Prof. Dr. Christoph Busch, Prof. Dr. Andreas Heinemann, and Prof. Dr. Marta Gomez-Barrero.
Abstract
Biometric recognition systems are part of our daily life. They enable a user-convenient authentication alternative to passwords or tokens as well as high security identity assessment for law enforcement and border control. However, with a rising usage in general, fraudulent use increases as well. One drawback of biometrics in general is the lack of renewable biometric characteristics. While it is possible to change a password or token, biometric characteristics (e.g. the fingerprint) stays the same throughout a lifespan. Hence, biometric systems are required to ensure privacy protection in order to prevent misuse of sensitive data. In this context, this Thesis evaluates cryptographic solutions that enable storage and real time comparison of biometric data in the encrypted domain. Furthermore, long-term security is achieved by post-quantum secure mechanisms.
In addition to those privacy concerns, presentation attacks targeting the capture device are threatening legit operations. Since no information about inner system modules are required to use a presentation attack instrument (PAI) at the capture device, also non-experts could attack the biometric system. Thus, presentation attack detection (PAD) modules are essential to distinguish between bona fide presentations and attack presentations. In this regard, different methods for fingerprint PAD are analysed in this Thesis, including benchmarks on several classifiers based on handcrafted features as well as deep learning techniques. The results show that the PAD performance depends on material properties of the used PAI species in combination with the captured data type. However, fusing multiple approaches enhances the detection rates for both convenient and secure application scenarios.
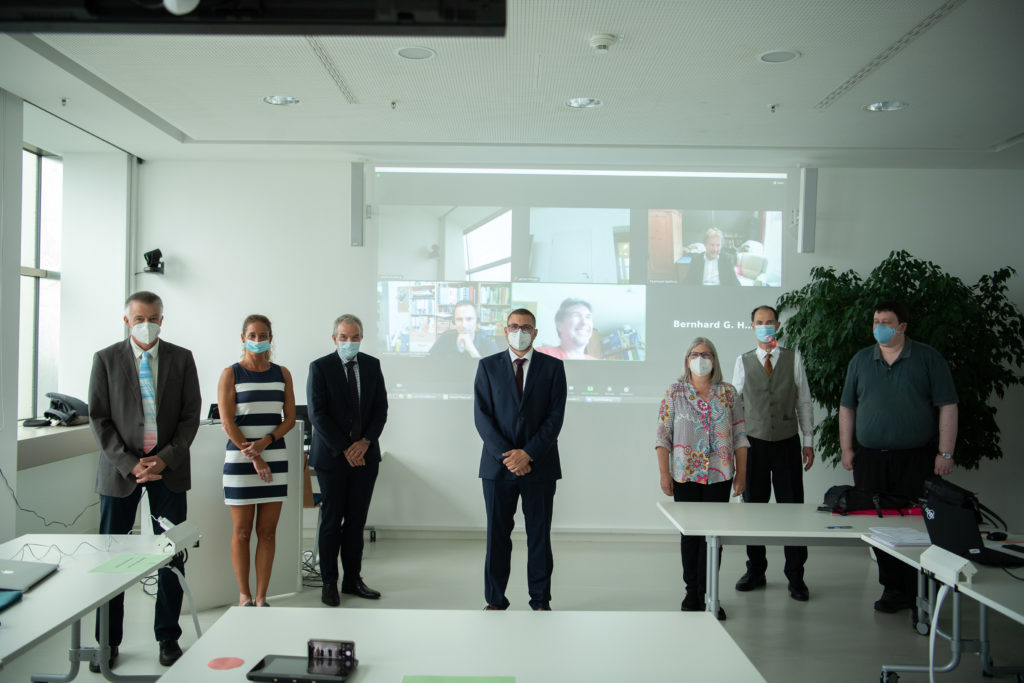